

The research community continues to develop better algorithms and larger models. New techniques, like diffusion models, shrink down the costs required to train and run inference. Wave 3: Better, faster, cheaper (2022+) Compute gets cheaper. Despite these limitations, the earliest Generative AI applications begin to enter the fray. They are large and difficult to run (requiring GPU orchestration), not broadly accessible (unavailable or closed beta only), and expensive to use as a cloud service.
SKYBOX STYLIZER CODE
OpenAI’s GPT-3 stands out: the model’s performance is a giant leap over GPT-2 and delivers tantalizing Twitter demos on tasks from code generation to snarky joke writing.ĭespite all the fundamental research progress, these models are not widespread. Between 20, the compute used to train these models increases by 6 orders of magnitude and their results surpass human performance benchmarks in handwriting, speech and image recognition, reading comprehension and language understanding. Sure enough, as the models get bigger and bigger, they begin to deliver human-level, and then superhuman results. All rights reserved SCIENCE.ORG/CONTENT/ARTICLE/COMPUTERS-ACE-IQ-TESTS-STILL-MAKE-DUMB-MISTAKES-CAN-DIFFERENT-TESTS-HELP Sources: © The Economist Newspaper Limited, London, June 11th 2022. As AI models have gotten progressively larger they have begun to surpass major human performance benchmarks.
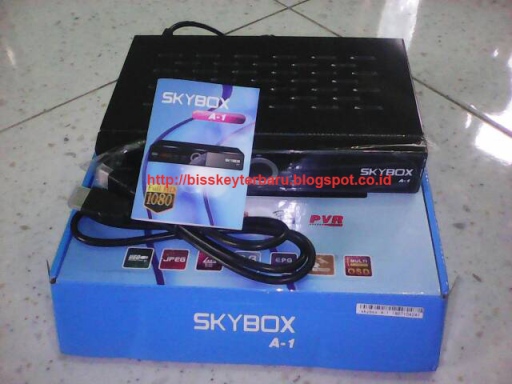
These models are few-shot learners and can be customized to specific domains relatively easily. Wave 2: The race to scale (2015-Today) A landmark paper by Google Research ( Attention is All You Need) describes a new neural network architecture for natural language understanding called transformers that can generate superior quality language models while being more parallelizable and requiring significantly less time to train. Generating human-level writing or code remains a pipe dream. However, they are not expressive enough for general-purpose generative tasks. These small models excel at analytical tasks and become deployed for jobs from delivery time prediction to fraud classification. Wave 1: Small models reign supreme (Pre-2015) 5+ years ago, small models are considered “state of the art” for understanding language. The category is changing faster than we can capture, but it’s worth recounting recent history in broad strokes to put the current moment in context. Generative AI has the same “why now” as AI more broadly: better models, more data, more compute. Therefore, Generative AI has the potential to generate trillions of dollars of economic value. Generative AI can make these workers at least 10% more efficient and/or creative: they become not only faster and more efficient, but more capable than before.

The fields that generative AI addresses-knowledge work and creative work-comprise billions of workers.

The dream is that generative AI brings the marginal cost of creation and knowledge work down towards zero, generating vast labor productivity and economic value-and commensurate market cap. Certain functions may be completely replaced by generative AI, while others are more likely to thrive from a tight iterative creative cycle between human and machine-but generative AI should unlock better, faster and cheaper creation across a wide range of end markets. Every industry that requires humans to create original work-from social media to gaming, advertising to architecture, coding to graphic design, product design to law, marketing to sales-is up for reinvention. Generative AI is well on the way to becoming not just faster and cheaper, but better in some cases than what humans create by hand. This new category is called “Generative AI,” meaning the machine is generating something new rather than analyzing something that already exists. But machines are just starting to get good at creating sensical and beautiful things. Up until recently, machines had no chance of competing with humans at creative work-they were relegated to analysis and rote cognitive labor. We write poetry, design products, make games and crank out code. This is called “Analytical AI,” or traditional AI.īut humans are not only good at analyzing things-we are also good at creating. Machines can analyze a set of data and find patterns in it for a multitude of use cases, whether it’s fraud or spam detection, forecasting the ETA of your delivery or predicting which TikTok video to show you next.
